What is AI’s role in shaping financial markets?
The below content is taken from the Intuition webinar ‘Balancing Act: AI’s Role in Shaping Financial Markets’ where learning solutions specialist Alastair Tyler, financial markets expert Peter Leahy, and AI thought leader Maury Shenk discussed the evolving world of AI in finance.
The full transcript can be seen below along with video clips of the various topics covered.
If you would rather watch the whole discussion, please click here.
Alternatively, you can listen to a podcast version of the discussion via the below MP4.
Contents
- Introductions
- The risks of AI in finance
- What is Artificial Intelligence? The differences
- AI risk and compliance in the middle and back office
- The future of AI
- AI taking over jobs
- The monotony of AI
- New skills for the AI world
- How should professionals be informed about the world of AI?
- Open source models and risk and governance in AI
- AI and bias in financial markets
Introductions
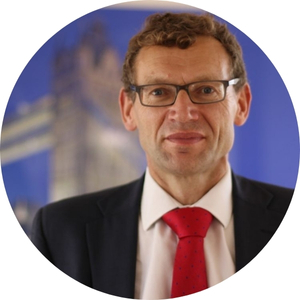
Alastair Tyler
Let’s make a start. And what I’d like to do really is ask our two panelists, first and foremost, just to introduce themselves. tell us a bit more about your background in finance, and also, of course, your interest in AI.
So, if I may, let’s start with Maury. Thank you.
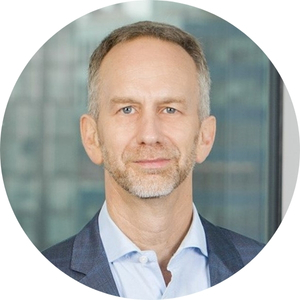
Maury Shenk
Thanks, Alistair. I’m Maury Shenk.
I do a number of things related to AI. I’ll mention two quickly. I’m founder and CEO of an AI enabled ed tech company called LearnerShape. And I’m also on the AI team at a global law firm called Steptoe, where I used to be a managing partner. I’m an advisor there.
In financial services, I speak regularly about implications of AI for financial services. This is one of them. It’s one of various AI sectors that I look at.
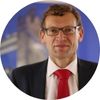
Alastair Tyler
OK, thank you, Maury. And over to you, Peter.
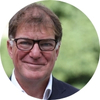
Peter Leahy
Hi, I’m Peter Leahy. I’m a financial consultant.
I specialize in fixed income and derivatives markets, touching on equity in some respects as well. My background is in dealer firms as a trader and salesperson. I’ve been a consultant for some 10 or 15 years now. And in recent times, because of my focus particularly on market analytics, I have found myself looking more and more at artificial intelligence and machine learning, because that is coming to the fore.
It’s an exciting development that holds out possibilities which are highly exciting and potentially profitable, such as better analytics and analysis of markets.
We must also always, of course, be mindful of risks and the potential for malfeasance. And that’s part of the discussion as well. Thank you.
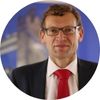
The risks of AI in finance
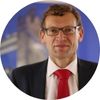
Alastair Tyler
I think AI is very prominent in the news today, both in a positive sense, as we’re hearing about the latest releases on GPT-4 and Google Gemini. So, it’s a fast moving area. But also, we’re hearing there is negative news stories and lots of concern surrounding AI, how ultimately it’s going to take over the world with deep fakes and fraudulent activities.
Is this true? Or how realistic kind of vision is this? Maury, what’s your view on this?
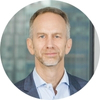
Maury Shenk
Well, I think AI has much more potential for benefit than it does for risk. However, that doesn’t mean we shouldn’t be concerned.
Airplanes have great benefits, but we still regulate them. And things still happen, as we’ve seen with Boeing. AI is the same thing. It will have great benefits. And there are some serious risks. Misinformation, as you mentioned, its effects on elections, potential of destabilizing effect in international conflict from autonomous weapons.
But in financial services, what we’re here to talk about today, there are some big risks.
Market manipulation through misinformation, instability of markets. We’re adding new technology that could lead to things like the flash crash that we had a few years ago, if we don’t properly govern it.
And then cyber security, which is very important for financial services. That’s a broader risk in other sectors, but I think it’s a significant one.
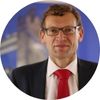
Alastair Tyler
Thank you for that.
And Peter, just from your perspective, as someone that’s obviously worked in the front office in financial markets for many years, what’s your view to start with just looking at it from a perspective of risk?
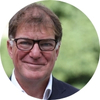
Peter Leahy
I think it’s important to recognize risks. There are important risks out there. And Maury just touched on what to my mind is, certainly from my background as a markets person, the potential for market moving and manipulative misinformation is something we must all recognize. And it’s important that we seek out ways to guard against that.
Some of the ways are as yet not obvious. We have seen in recent times, I can think of an example recently, there was a terrorist attack in one country, who were anxious to accuse, I’ll leave names out of this, another country. And we quite quickly had the Defense Minister of the second country, kind of owning up to it. Until later, it turned out that that wasn’t a genuine news piece at all. We have no idea who was behind it. But it just shows you that there are avenues that we need to be careful we don’t go down or fall into those kind of traps.
A lot of what I’m here to talk about today is in the field of machine learning and how that can be an adjunct and means to, if you will, leverage human skills in market analytics. I think there are fewer ethical concerns there than perhaps there are in the field of impersonation and creating fake news and so forth, which is what Maury’s just already touched on.
What is Artificial Intelligence? The differences
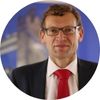
Alastair Tyler
That leads nicely into our next question.
This is a whole new world for all of us, so we’re having to come to terms with, a bit like in finance in a sense, with new terms, new expressions, and there’s plenty of scope for confusion across people using terms when they don’t actually strictly apply.
So, if I may, it would be great if you could, Maury, perhaps help us here to explain the difference between chat GPT, generative AI, and machine learning. And also, why is it so important that we understand the difference, particularly, say, if you’re a regulator or a financier? Thank you.
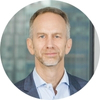
Maury Shenk
I may leave some of the details of machine learning to Peter, he’s been looking a lot at that. From a historical perspective, the term AI was invented in 1956. And people at that time thought within a few years that we would have a solution to having computers act like humans. That obviously didn’t happen.
And AI went through a period of, we call them ‘AI winters’, where people thought AI didn’t have promise. And for many years, decades, literally, AI was something else. It was what we called expert systems or good old-fashioned AI, where we tried to write down the rules how a computer should behave to be artificially intelligent.
That didn’t work very well. And when AI finally started to work was around 2012, when we started to use machine learning, where the program would learn from the data rather than being given explicit instructions to deep learning large neural networks, to first recognize images, do automated translation, automated fault detection, things like that, that has had a huge impact in industry. And I call that predictive AI, it’s predicting whether two words are the same in two different languages, predicting whether an image is a cat or a dog, or a building.
And then later in the twenty teens, we started to come up with generative AI. So the ability to use AI to generate new content.
And that’s what ChatGPT and these LLMs are. Generative AI has been making an impact for four or five years, but only in the last year and a half with the release of ChatGPT has it come to everybody’s attention.
Those are the two big categories I think people need to understand.
- Predictive AI, which is still the most important in financial markets for sure.
- And then generative AI, ChatBots, ChatGPT, etc.
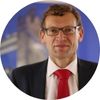
Alastair Tyler
Fantastic. Over to you, Peter, just to build on that. And in particular, say a bit more about machine learning and where its role and where it fits in alongside AI.
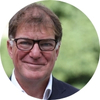
Peter Leahy
Machine learning has potentially enormous roles to play in the analysis of markets. I’ll just start with a couple of broad categories of AI.
If you ask for a categorization of it, you’ll hear terms like supervised learning, unsupervised learning, reinforcement learning, and so forth. We’re not here today to worry too much about those, but certainly learning from data and from market activity is one thing, because that allows you to do two things.
One is to seek to understand, okay, if these things happen, and a lot of these are real life in markets and human experience, is never quite as linear or if you will, single factored.
A lot of our models, for example, option pricing using the Black-Scholes model, we’re talking about factors moving in a linear way and only really looking at one changing factor at a time.
What we can potentially do or get from machine learning is to try to understand, go back over data from the past and try to understand what factors appear to have been influential or important. Secondly, what weightings to apply to those factors, and we can begin then to seek to model and to understand how the dynamics of a market are actually operating.
A second branch of machine learning would be reinforcement learning, where rather than trying to define the dynamic of how this data impacts this market price, for example, what we might do with machine learning, is we run through a decision tree. Think of one of those decision trees we all see, if you want to do this, yes or no, and then you move on to the next plot, not dissimilar to that, a decision tree here that says, okay, we want to, for example, maximize wealth by buying or selling this small set of things. And we can iteratively try out decision makings to see how that impacts the total value of our trading book or portfolio. And of course, from that then, rather than seeking to understand a formula or a model, we’re trying to learn behaviors which appear to be successful given an objective such as, as I said, the value of a trading book or portfolio.
In short, what we’re trying to do, particularly with machine learning, is to find powerful ways to leverage the kind of things that human beings, the logic and the motives and the methods even, that human beings have been applying far slower to markets and for a long time.
AI risk and compliance in the middle and back office
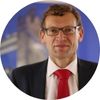
Alastair Tyler
Thank you.
If I may, because there’s opportunities, it’s such an important area, we want to broaden that a little bit further.
So far, we’ve looked at some examples from the front office. I’m interested to find out a little bit more about what’s possible now to improve the way that banks and investment firms are operating in from the middle and the back office, looking at the risk operations, compliance areas of the business, which obviously are so important.
Who would like to respond on that one?
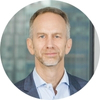
Maury Shenk
I’m happy to respond. I think the predictive tools that I mentioned that have been around for quite a while are useful in compliance. For anti-money laundering compliance, for recognizing anomalies, for cybersecurity, for a lot of those things, being able to do human tasks where a human recognizes something, but do it more efficiently with the tool is a very powerful application.
And there’s a lot of regtech companies that have been around for quite a while that are using some traditional data science methods as well as AI to do those things. I also think that generative AI is quite risky at the moment, particularly for regulated sectors like financial services, because it hallucinates and it says things that are wrong. And there are plenty of stories out there about people tricking chatbots into selling them a cheap airplane ticket or something like that.
That’s a pretty big risk in financial services, but we will get better at that. I think at least in low-risk applications, there are a lot of opportunities to use chatbots for a variety of purposes, although that is not as much back office.
To go beyond that, what people are excited about in the last couple of months is what they call ‘agent workflows’, where an LLM, a generative AI agent that can respond to commands, goes off and does things for you.
And we expect there to be lots of these around. And these things can use existing tools out there. That is a huge area for expansion.
I think there’s a great deal of unknown, where that will lead us, but I think people are going to figure out how to use these generative tools to go out and act as independent agents and use a whole host of tools, back office and front office.
The future of AI
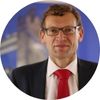
Alastair Tyler
So just building on what you’re saying there, Maury, around future opportunities, how much of our working day in financial services will be spent working in collaboration with these AI tools? At the moment there are all sorts of systems and tools that investment firms use, how much do you think it will start to overtake or become the dominant way that we’re doing business? Or do you think that’s perhaps exaggerating its importance?
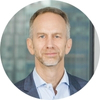
Maury Shenk
Three to five years is an interesting time frame. I think it was Bill Gates who said, we overestimate change within a year to two years and underestimate change over a ten year timeframe.
But over the five to ten year timeframe, I think we’re going to see huge impact of AI.
AI is one of those rare general purpose technologies, like fire, or steam power, or electricity, or digital computing, that operates across sectors, and people are just figuring out how to use it.
The short answer is in ten years, I think we will see lots of impact. And I don’t know where it’s going to be. It’s kind of fun to live in these times and watch that.
AI taking over jobs
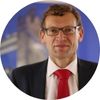
Alastair Tyler
Perhaps we should address the elephant in the room. What does this mean about jobs?
Is this going to be such that it becomes so efficient and dominant that we don’t really need the roles that we’ve created and, will many of the roles in the front, middle, and back office, will they become redundant?
What’s your view of how this tool will change the way financial services firms are structured?
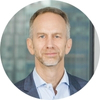
Maury Shenk
There has been predictions for the past ten or fifteen years that AI is going to take all of our jobs.
Evidence from previous technological revolutions is that they do not, they create a net increase in employment. And so far, there is significant evidence that that is what AI will do as well. There certainly hasn’t been wholesale job loss, but there’s also huge disruption.
A lot of jobs will change, jobs will be eliminated and new jobs will be created. I think financial services is one sector where people are going to be doing different things, and they’re going to have to be retrained to do those different things.
There’s a knock-on effect, which is social inequality. The Industrial Revolution was a time of great growth in jobs, but it was a horrible time for social equality, because these new technologies tend to reward capitalists. And that’s something we have to figure out a way to deal with as a society.
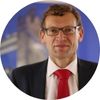
Alastair Tyler
Indeed. And Peter, do you want to comment on this as well?
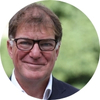
Peter Leahy
Just following on from what Maury was just saying, I have read somewhere in the last few days that there are fantastic salaries being offered for people who are able to write code and so forth at the sharper end of AI and machine learning. So what Maury was saying about equality and so forth is germane to that discussion.
Just on the other point that was being made there, the way I see it is that certainly, as I’ve said, I’ve got a lot of interest in market analysis, and my view is that I don’t see AI making people redundant.
I see AI and machine learning, which is my particular area of interest in that, I see it as making people better at their jobs. It leverages skills, it gets their skills more powerfully applied.
One little area which we could talk about, we’ll talk about equities in a moment, but there’s a fantastic number of people, particularly in US markets, concerned with trying to predict mortgage prepayments. That is a field that’s absolutely cut out for AI and particularly for machine learning, because you’re talking about stuff which has got financial logic to it, but also trying to interpret the vagaries and complexities of human behaviour. You set a machine to go and learn not just the financial stuff, but some of the human behavioural dynamics, and you get something which is highly valuable. I still don’t see how that makes a machine replace a human analyst.
It just makes the human analyst better equipped.
The monotony of AI
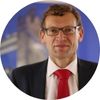
Alastair Tyler
Here’s an interesting question, which I’m sure I know both of you gents have quite firm views on.
If everyone applies AI using the same tools, will we end up in the same place in terms of stock picking, investment decisions, etc?
I can see you smiling there, Peter, so over to you.
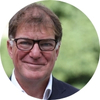
Peter Leahy
Well, I don’t believe that for a moment. I think that is just scare stories from, I want to say from some financial journalists, but I’m well aware that some of that has been put down as quotes from the chair of the Securities and Exchange Commission in the States. He is somebody whose job is to make us aware of risks and problems.
However, we’re talking here about tools. Tools get used by human beings. As Maury was saying, it’s an aeroplane. I use a similar idea. It’s a motorcycle, which is a really useful and beautiful thing, but they can be lethal. Same idea.
The way I see it, AI is going to be used, is already being used, by different analysts in slightly different ways and with different specifications, which means that we don’t all expect that we’ll reach the same conclusions about, for example, and I know that Chairman Gensler used the idea of stock selection and stock screening, we don’t all end up with the same short list of stocks because we specify what the computer needs to be doing in slightly different ways.
Even if we did end up with the same short list of stocks, bear in mind that an equilibrium market price is where the power of sellers has found a force equal to the power of buyers. That will always be the case. Some will believe and have more confidence in the AI advice or results than others.
New skills for the AI world
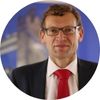
Alastair Tyler
What are the key skills that people working in financial services need to develop in this whole area?
Maury first, if I may.
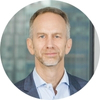
Maury Shenk
I think that there are three areas.
One is to have a general understanding of the kinds of AI and what they can do.
You get an intuition about whether using AI in a particular circumstance makes sense. Obviously, the level of depth of that understanding will depend upon the role you have.
The second is understanding the risks.
We started off talking about how AI has bigger opportunities than risks, but we need to look out for the risks, know what to do, know what not to do.
Then third, depending upon your role, again, have some basic practical understanding of how to use these tools well.
A lot of companies are making chat GPT, often an internal version of chat GPT, or some similar tool available to their employees. Understand how to use these tools well. There are some techniques of prompt engineering where you can get better answers if you know them well.
I would say those three areas.
How should professionals be informed about the world of AI?
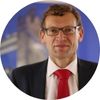
Alastair Tyler
Peter, I know you’ve been busy preparing some learning materials in this area, so good to get your view on this as well.
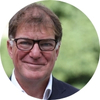
Peter Leahy
I’m going to say that I’m now answering, if I may suggest, a slightly different question to the one that Maury just gave an eloquent and very interesting answer to, which was more about how should professionals be informed about the entire area.
My focus has been on how to apply some of the subsets of AI, particularly machine learning, in markets. I guess a small plug here for Intuition’s Know-How product. I have been commissioned to write three things.
One on the application of machine learning in equity screening, so stock picking, if you will.
A second one on the application of machine learning in fixed income. Within that, talking about identifying yield curve shifts that are less or more likely filtering out anomaly trades for those that have a realistic profit potential.
As I’ve already referred to, analysis of mortgage-backed securities and prepayments.
There’s a lot to be said about those three areas.
The third topic that I’ve written about in the Know-How catalogue is on the use of machine learning, particularly reinforcement learning, so-called, in the context of trading and hedging derivatives. Within that, most especially options. That’s, again, a fascinating field because we all know that some of the models we use, most notably perhaps Black-Scholes, have significant shortcomings. They focus, as I’ve mentioned already, too heavily on simple movements and are often rather wedded to normal distribution and for a limited, or even in some cases, single dynamic factor.
By applying machine learning to derivatives, we’re able to actually move that whole area of analysis a whole few steps forward, which is exciting.
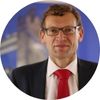
Alastair Tyler
No, that’s great. No, thank you, Peter.
I think one thing we can predict is that this is an area where there are, by the nature of it, because it is, we’re all kind of relatively new in terms of experience, so there are going to continue to be new learning programs from organizations like Intuition and keep an eye on our, as Peter was saying there, Know-How tutorials and future updates on that.
Open source models and risk and governance in AI
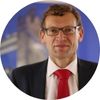
Alastair Tyler
So, here’s a question that’s come in. Will the risk elements linked to using AI for financial services be mitigated with the open-source models which can be adopted? And what would be the governance around them?
So, if I may, Maury, what’s your view on this kind of whole area of risk and governance?
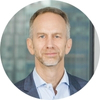
Maury Shenk
The question was about open-source models, and I don’t think that open-source models have a huge effect on risk management. I think they’re a useful additional tool.
People are worried that open-source models are going to spread the capabilities of AI to people who shouldn’t have it. But in financial services where you’re making a deliberate decision to use a model or not, I think you’ve got to put similar governance structures around open-source and closed-source models to decide what data you put into them, what governance structures you have.
There are slight differences, but I don’t see open-source as hugely linked to the risk problem.
AI and bias in financial markets
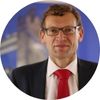
Alastair Tyler
Okay. And I think we’ll have to make this one our final question.
Will it not be more difficult to gauge if AI models are biased as compared to other models?
Peter, what’s your view around being able to identify the bias? To what extent they’re more susceptible?
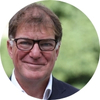
Peter Leahy
I think with what I was talking about in terms of the markets I mentioned, I think we need always – and in machine learning and everything I’ve understood about it, people are mindful of periods of time, and we all know that in one period of time, one moment in history, the dynamics or the influences on markets are another set of things from what they will be at another moment.
Right now, for example, in a lot of markets, we’re pretty obsessed by inflation numbers. That isn’t going to always be the case. So I think that a lot about, for example, the use of machine learning is about recognizing biases and actually exploiting them. I don’t think a correct application of any of these techniques should leave the analyst wide open to being hoodwinked.
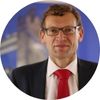
Alastair Tyler
Great, thank you. Sadly, we had all too short a time, but I’m confident this is a topic we will come back to again, possibly both through this kind of format, but also through other learning sources that we provide for our clients.
I want to extend a big thank you to both Maury and Peter for all your insights, and again just to remind everybody we will be sending around a recording of today’s session for you to view and share with your colleagues.
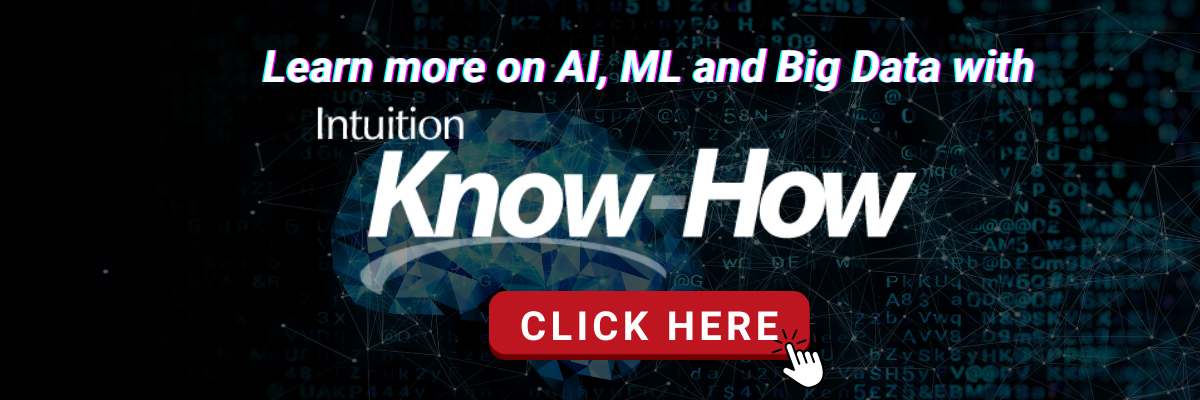