Finance reimagined: Mastering machine learning
The advent of machine learning (ML) has ushered in a new era in the financial industry, prompting a significant shift from traditional methodologies to sophisticated data-driven systems.
This article presents a comprehensive understanding of the crucial role machine learning plays in finance, emphasizing the necessity for finance professionals to embrace this technology to successfully navigate an increasingly digitized financial landscape.
[How to leverage data within your corporate compliance program]
Machine learning: Transforming the financial landscape
The inception of ML has marked a seismic transition in the financial sector.
What began as rudimentary exercises in algorithmic trading has evolved into complex artificial intelligence systems that have become an integral part of financial operations.
The potential of these technologies is seismic, with significant improvements in efficiency, accuracy, and innovation.
Key applications of ML in Finance
Machine learning has several applications across the financial spectrum. In algorithmic trading, it provides effective market predictions and trading strategies.
It has revolutionized risk management through predictive models that facilitate the assessment and mitigation of risks.
ML also plays a crucial role in detecting fraud and bolstering cybersecurity efforts, safeguarding financial assets, and data.
Furthermore, it enhances personalized banking services, offering tailored financial advice and product recommendations to customers. Regulatory compliance and reporting have also seen increased efficiency and accuracy with AI-driven systems.
[Transforming L&D: Mastering learning analytics for real world impact]
Key Applications of ML in Finance
Implications for finance professionals
The proliferation of ML in finance has significantly reshaped job roles and skill requirements. Professionals are now expected to adeptly blend financial acumen with technical proficiency, particularly in data literacy and analytics.
These changes pose both challenges and opportunities, demanding adaptability but also offering avenues for career growth and specialization. For instance, roles like data scientists and AI specialists in finance are gaining prominence, reflecting the shift towards a more technologically adept workforce.
The importance of learning machine learning
For finance professionals, mastering machine learning has transitioned from being optional to indispensable. It equips them to stay competitive in an industry characterized by rapid technological advancements.
By leveraging ML, professionals can make more informed decisions, drive innovation, and enhance customer satisfaction. Furthermore, understanding these technologies is critical to prepare for future developments and their potential impact on the financial sector.
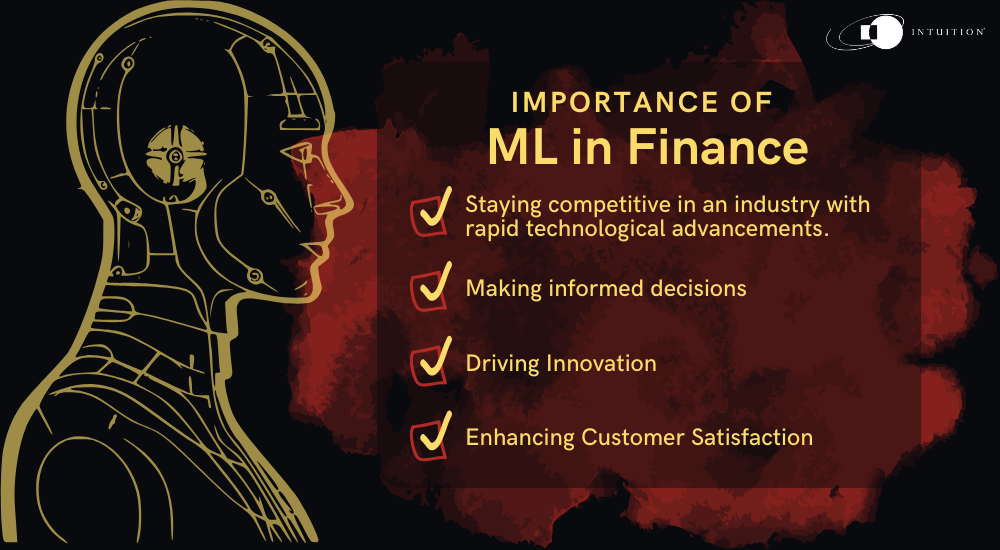
Resources for learning ML
To adapt to this new paradigm, finance professionals can tap into various educational resources, from online courses to specialized training programs. These resources provide essential knowledge in machine learning techniques and their application in finance.
Learning from peers and industry leaders who have successfully integrated machine learning into their practice can offer valuable insights. Incorporating these technologies into existing business practices and fostering a culture of continuous learning within organizations are also vital steps in this transition.
ML: Shaping the future of Finance
Looking ahead, machine learning is poised to further revolutionize the finance industry. As technology advances, we can anticipate more sophisticated applications, possibly reshaping the very nature of financial services. However, this evolution brings with it ethical and regulatory challenges, as the line between automated decisions and human oversight becomes increasingly blurred. Finance professionals need to be prepared to navigate these complexities as they arise.
Conclusion
In conclusion, the integration of ML into finance is not just a passing trend but a fundamental evolution of the industry. For finance professionals, an understanding of machine learning is crucial to navigate this new landscape effectively. As the sector continues to evolve, those who embrace and adapt to these changes will find themselves at the forefront of a more efficient, innovative, and data-driven financial world.
Frequently Asked Questions
Q: What is the difference between machine learning and traditional methods in finance?
A: Traditional methods in finance typically involve manual analysis of data and the use of pre-defined rules and models to make decisions. Machine learning, on the other hand, uses algorithms to identify patterns and connections in large datasets automatically.
Q: How is ML used in finance?
A: ML is used in finance for various tasks, including fraud detection, automated trading, anomaly detection, robo-advisors, tax optimization, risk management, and more.
Q: What are some potential drawbacks of machine learning in finance?
A: Potential drawbacks include the potential for bias in the data or algorithms used, complexity of models for new users, risk of errors or inaccuracies, and the cost of implementing ML in finance.
Q: How can financial institutions ensure that their use of machine learning is ethical and responsible?
A: Financial institutions should carefully consider the potential biases and implications of the data and algorithms used, regularly monitor the performance of machine learning models, and update them as necessary to ensure accuracy and relevance.
Q: What are some emerging trends in machine learning in finance?
A: Emerging trends include the use of neural networks, deep learning, reinforcement learning for more advanced and complex tasks, natural language processing for sentiment analysis, and Gaussian processes for improving risk management and decision-making in finance.
Browse other articles in this series:
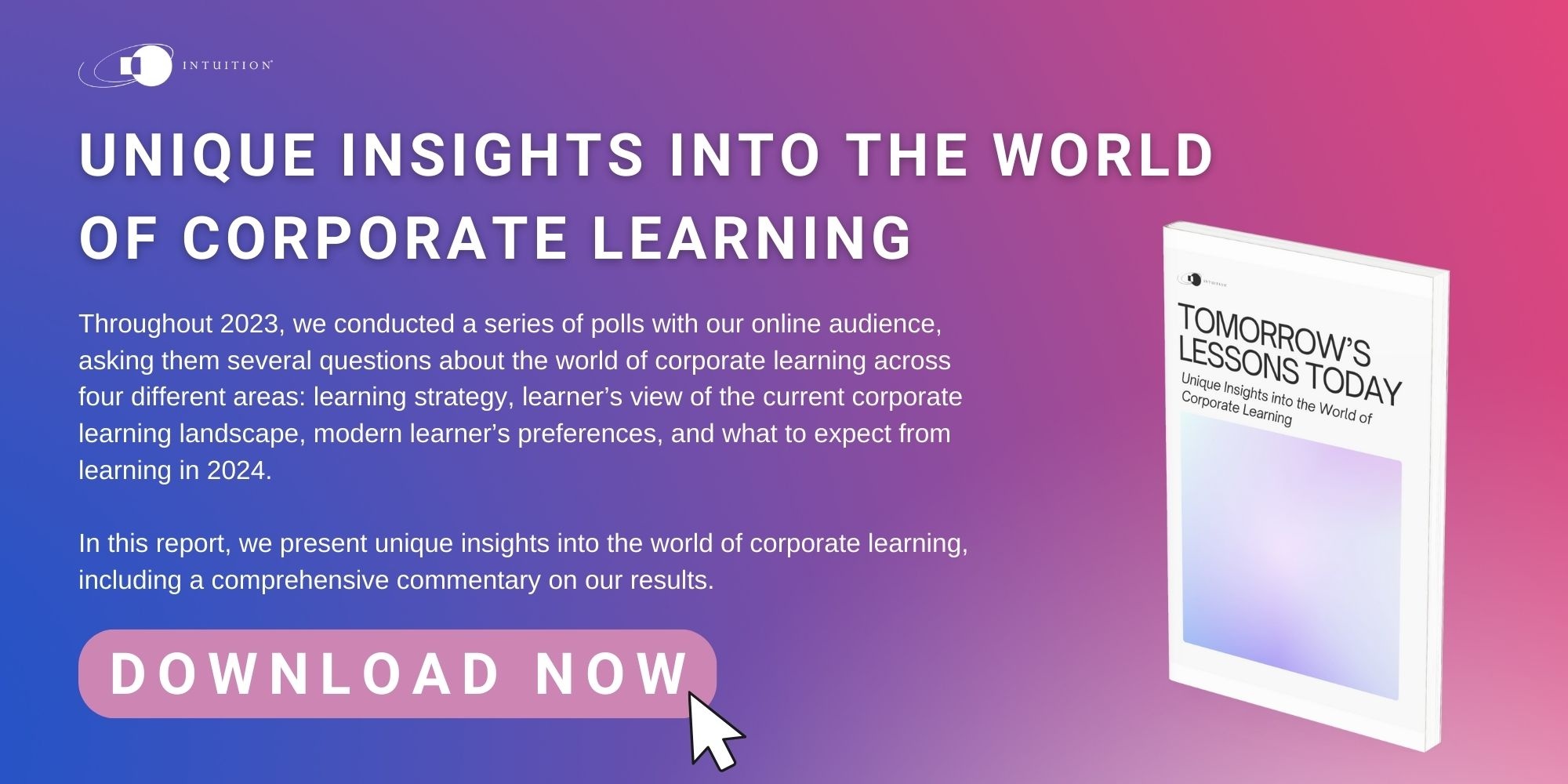