Big data defined: IoT, three Vs, uses, role in AI
Big Data has become a major buzzword in the past few years as we have collected more and more information about a wide range of objects, processes, people, and things.
This is due to both the increase in available computing power – processing and memory – on the back of the advent of cloud computing, and the interconnectivity of data-gathering sensors through the internet, known as the Internet of Things (IoT).
What is IoT?
The Internet of Things (IoT) refers to the network of objects and devices that are connected to the internet and embedded with sensors, software, and other technology so that they are able to gather and share data with other devices and systems.
For example, many homes today are equipped with smart thermostats, which monitor environmental conditions such as the temperature and report the information they gather to their owners via a mobile app. The owners can then manually turn on the heat or air conditioning, or remotely program the thermostat to keep the temperature in a given range. The parent company that produces the smart thermostat may also gather data about temperatures and settings – that information may be helpful to power companies by giving them insights into when demand is likely to spike.
Three Vs of Big Data
These two aspects of how the internet has developed in recent years – the availability of greater volumes of data and more computing power – have led to a surge of interest in Big Data.
But what exactly is it?
The original definition of Big Data states that it is defined by three “Vs”:
Volume
Big Data consists of a lot of unstructured data.
For some organizations it can be terabytes (TB) per day; for others, it can be much more. In 2018, for example, Walmart estimated that it collected 2.5 petabytes (PB) from 1 million customers per hour (1 TB = 1,000 GB = 1 million MB. One petabyte is 1,000 TB. So, 1 PB = one thousand million megabytes or 1015 bytes – a large volume of data indeed).
Velocity
Large amounts of data alone are not enough to qualify as Big Data, but if the data is also arriving fast, then it certainly qualifies.
The speed of Big Data also has implications for the design of any system that runs off this data.
Variety
This refers to the fact that the data may be unformatted and may encompass measurements, text, audio, and video, and direct feeds from sensors, which could be images or tabular data.
Using data that arrives in many different formats to generate actionable decisions requires a system that can manage all this information correctly.
A corollary of these three Vs is that Big Data is data that is too big to use in a normal way. It is not possible to look at Big Data in Excel or on a simple chart – it is too complex. It is not possible to make any inferences from Big Data without using special data tools to organize it and identify the patterns or structures that lie within.
The definition above has its limitations – it does not reflect the usefulness of Big Data, for example. To capture that, we must add two more Vs:
- Value: The data coming in may be big and fast, but does it contain any information of value? Walmart could, for example, easily record the height of everyone visiting a store with a simple optical sensor at the door, but would that data have any value for them?
- Veracity: The final V for useful Big Data is its veracity or truthfulness. Scraping social media feeds to gain insight into political preferences is a risky business as the truth of peoples’ intentions is easily disguised or overridden by automated scripts, bots, or malicious actors.
Armed with these five Vs, we can define what Big Data is. But what is it for and how do we use it?
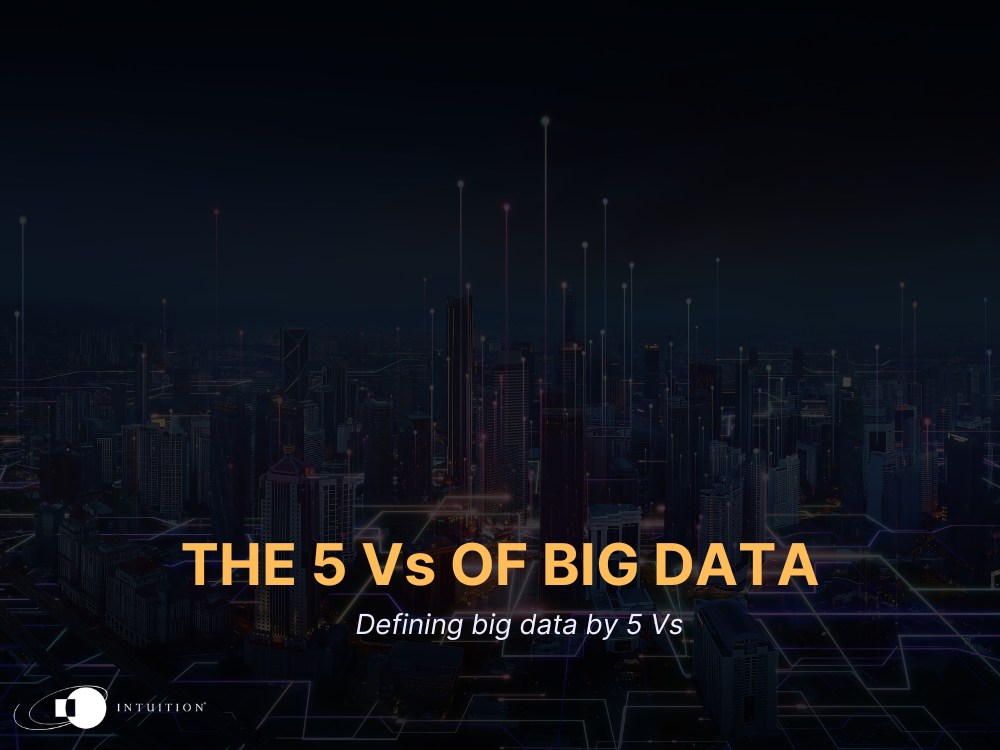
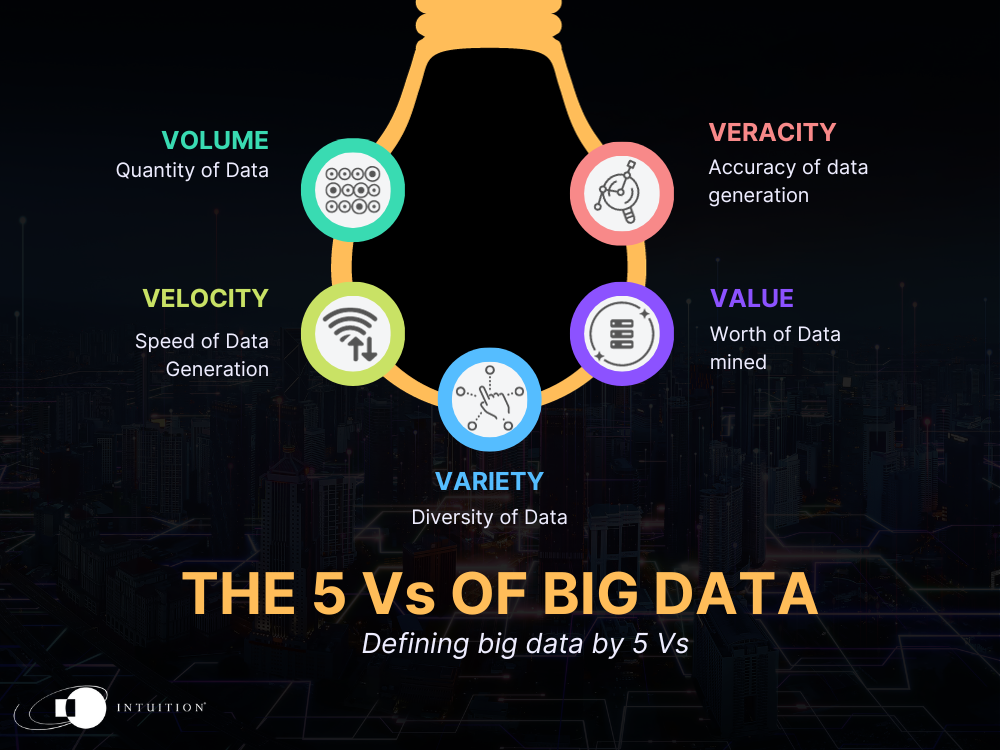
Test Yourself: Answer the Question Below –
How to use big data
[Finance reimagined: Mastering Machine Learning]
The complexity of Big Data means that we must take great care in managing and maintaining it.
The journey from “Big Data” to actionable information is fraught with pitfalls that can derail an organization’s best efforts.
With that in mind, here is a simple checklist of how to use Big Data effectively.
First, an organization must gather the data.
This includes understanding how it was sourced and what it truly represents in the real world.
Second, it is necessary to organize the data.
In many cases, this means filtering it to reduce the total amount and creating chunks of data that can be processed in batches.
Batches are necessary because it is very rare to operate continuous Big Data applications – the problem is generally too complex.
Third, the organization must understand the data.
This means developing a heuristic understanding of it, and then applying mathematical processes – such as a statistical operation – to extract actionable inferences.
It is important to understand the data before analyzing it to avoid the pitfalls of spurious pattern matching.
Finally, it is necessary to govern the data.
An organization must set up rules for access, and for adding new data so that the data remains consistent over time.
Without consistency, it is possible to see a pattern in a dataset that comes not from a real-world change, but from a change in data entry.
For example, upgraded sensors may lead to a totally spurious inference if the new data is processed along with existing datasets.
Similarly, without due care and careful governance, data may be lost or corrupted, again leading to false inferences.
Taking an organized approach to using Big Data is the best way to generate meaningful inferences.
AI & Big Data
[How computers add value to business: Modeling & Decision-making]
It could be argued that properly executed AI would remove the need for the data-wrangling described above and, in particular, the need to understand the data before analysis. The idea would be that an AI system could manage the data itself – it could organize it and structure it in useful ways before analyzing it.
While this is an interesting notion, it remains to be proven in practice. In reality, most AI systems require data that is carefully prepared and structured in ways that the system can understand. This is one of the reasons that much-anticipated AI applications such as driverless cars operating in real cityscapes with pedestrians, dogs, and cyclists are still a long way off.
Test Yourself: Answer the Question Below –
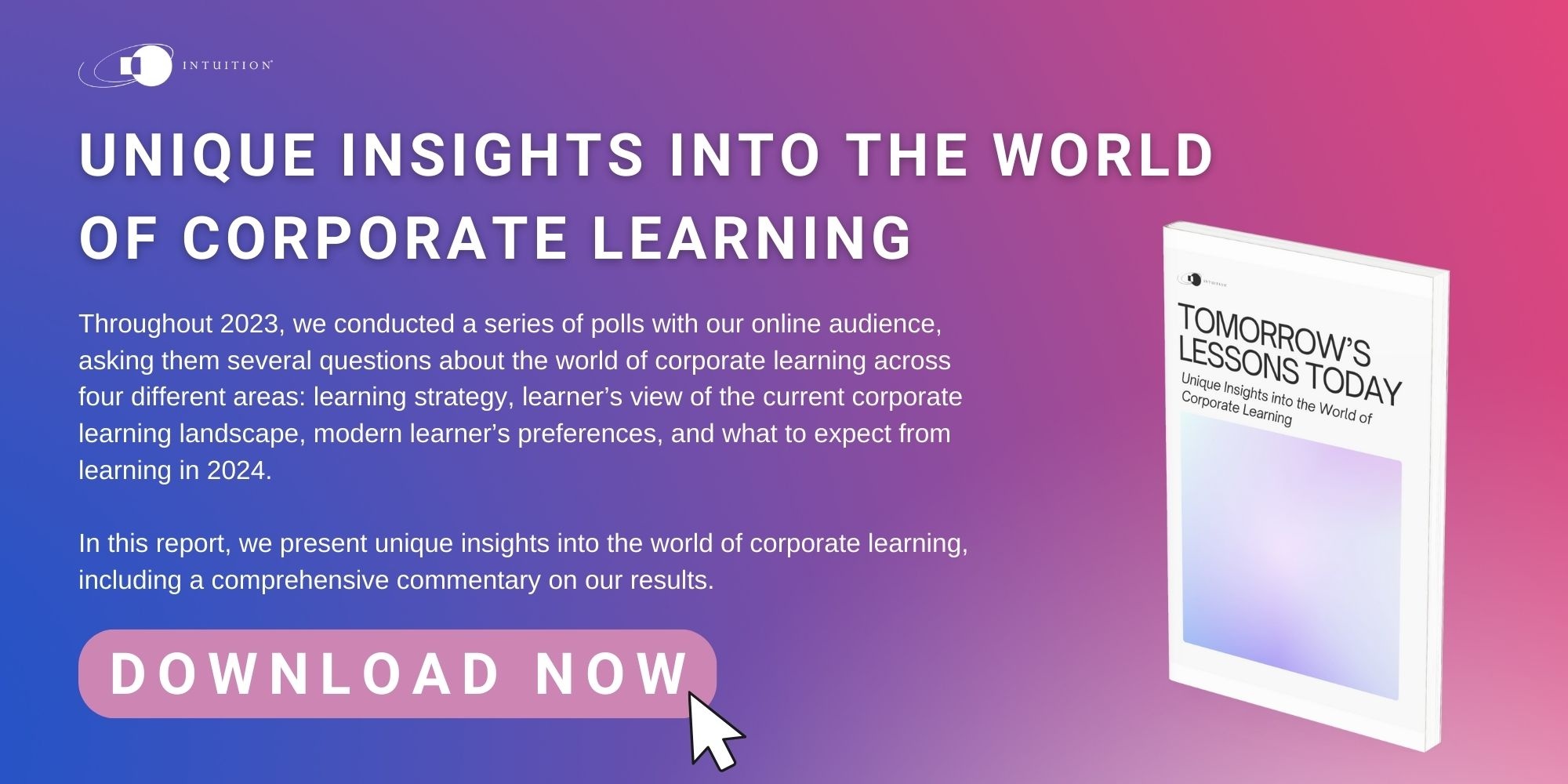